Human-AI Teamwork
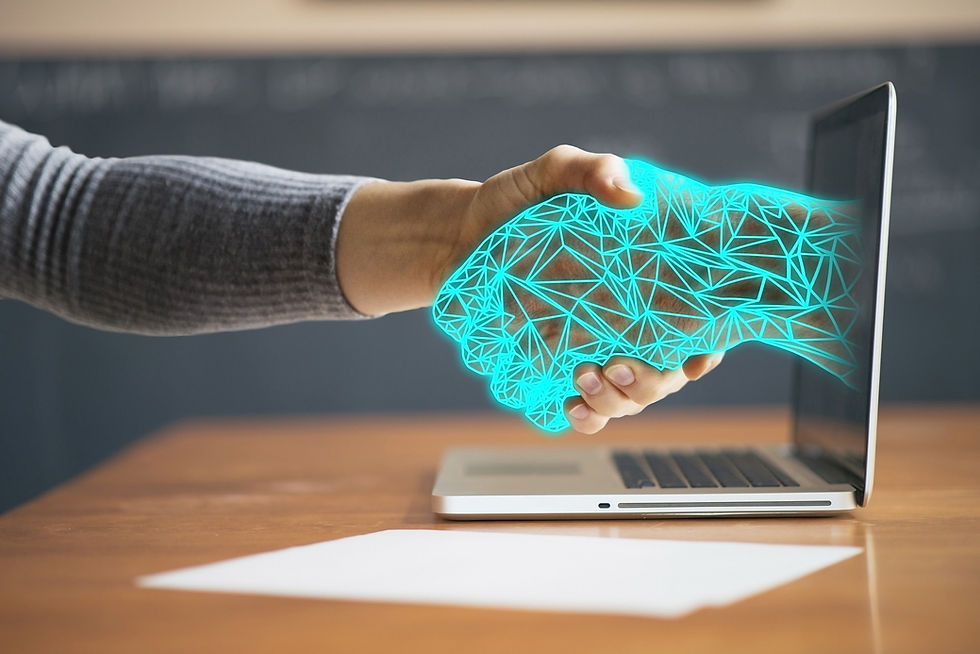
In the near future, the probability we will all be working with artificial intelligence (AI) to get our jobs done is a certainty. Yet we learn about working with people, but not with AI. How do we plan for better performing human-AI teams?
What is a Human-AI team?
This is a team that is composed of humans and AI that work together on various tasks. Here it is important to mention that AI is a complex system of hardware, software, data, and human ingenuity (maybe represented via a company) behind the scenes.
Human-AI roles in decision-making.
The Human-AI may work as part of larger, more complex system (car production lines, smart cities) or just operate as a team of two (for example, your financial analyst who depends on a software analysis package). Still, the important thing is to understand the role of AI in the work environment. Gartner has looked at the roles of humans and AI in decision-making. There are three scenarios:
Decision support: Decisions made by humans using data & insights provided by AI.
Augment decision making: These are hybrid decisions, with three scenarios:
a. AI suggests; human decides.
b. Human suggests; AI decides.
c. Humans and AI decide together.
3. Automated decision making: AI will make decisions with or without human oversight.
These levels of hybrid decision-making are illustrated below:

Characteristics of High-Performance Teams
To answer this question on characteristics of high performance teams, you must first look at what makes exceptional high-performance human teams. Two years, 200 interviews, and 180+ teams later, Google (now Alphabet) found these five characteristics:
Psychological safety: The ability to take risks on the team without feeling compromised? (Important, it is the feeling! AI has no feelings so the question is how does AI make us feel?)
Dependability: The ability to rely on each other to do high-quality work on time (AI always can process data faster than a human, so how does an organization appreciate the uniqueness of what a human can bring to the team).
Structure & clarity: The organisational goals, roles, and implementation plans clear to all members of the team? (AI does just what it is programmed to do - so the burden falls on the human).
Meaning of work: The personal interpretation of work and its importance for each of the team members. (AI does not have a personal interpretation so again how does the AI affect the human?)
Impact of work: The belief that the work we’re doing matters in the larger scheme of things. (AI cannot have beliefs so how does the AI influence a human's belief?)
In the above study, Google then continued to look at psychological safety and found those teams which began every team meeting by sharing a risk taken in the previous week, saw a 6% improvement in psychological safety and 10% in structure and clarity ratings. The issue is that AI cannot do this unless it is transparent and the extended teams discuss insights, failures and positive cases.
Challenges for Human-AI teams
As you can see from my comments above, the challenge of creating psychological safety (assuming the other points are a sub-set of the first) is a big one.
There are several issues that need to be unpacked.
1. Awareness: Is the employee aware of how the system works, and were they consulted in its development? Very often, since companies outsource, this is not the case—this causes either mistrust or demotivation (both of which are not good for AI-human teams). Here we need transparency in the design process, some oversight of data fed into these AI systems, and a strong feedback loop on insights and performance of the AI system. In my experience, senior management often does not understand AI systems. They then make poor decisions based on opportunities without understanding the risks (short and long-term) to the organization's performance and culture. It results in reduced capabilities and AI systems designed for different contexts being forced to work in less-than-optimal situations.
2. Training: Senior management/IT departments sometimes deploy systems without adequately training employees. Training is critical as it builds trust. Every update of the AI system should be communicated in simple language. This communication aims to let employees know why (including what is not working) and what these updates should now achieve. This process means embedding transparency and making explicit the knowledge and team goals. These discussions also feed into performance appraisals.
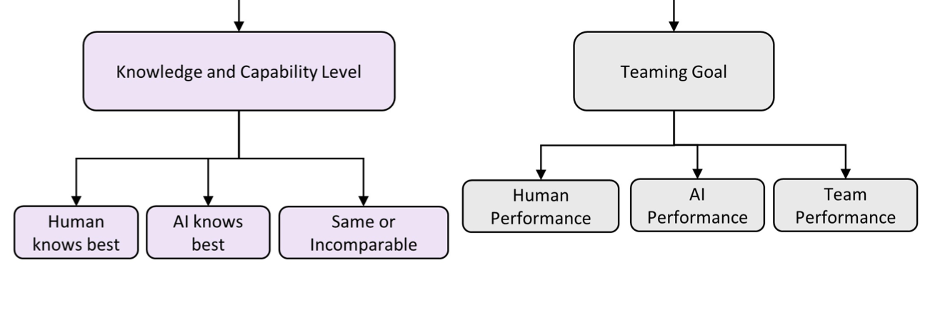
3. Accountability and Responsibility Structures: Management adopts AI systems to deliver a certain level of performance. When there is a failure, there needs to be accountability for the failure and responsibility for their actions or lack of actions. AI often functions backstage and behind the scenes, which makes this very murky to assign accountability. Yet management that adopts, endorses, funds, and deploys an AI system must be clear on the structure of decision-making and the risks of delegation to AI. The responsibility cannot be the vendor alone, as the vendor was approved. What should senior management do? Design strong policy guidelines and ensure that the governance of AI systems is world-class. Look at vendors' experience and their governance policies (AI and data). This is critical. Make sure you budget not just for new projects but their maintenance and upkeep and continuous training of all employees.
4. Human-in-the-Loop: I cannot stress this enough. Make sure that in executing complex decisions using AI, that human judgment and experience have a method to bypass any automated AI decision-making. The biggest failures of AI – the 2022 UK Post Office Scandal, the 2018-19 USA Boeing 737 MAX plane crashes, and the 2020 and 2022 Flash crashes of stock markets, are all recent examples where humans were considered inferior to the AI systems. But, more importantly, they could not bypass the decision-making chain of command in the time frame required.
We are at a tipping point. Gartner says that by 2025, 95% of decisions that use data will be at least partially automated. Maybe AI works well in narrow tasks, but as we start employing AI in more decisions at various levels, we will:
1. Lose human capacity and skills (which means if, for some reason, AI becomes inoperable, we may not have the backup skills needed). We already see this in our need for legacy system coders (older programming languages).
2. We are creating unemployment, job security fears, and greater distrust. The future is what we shape it to be. One of the Universal Human Rights is the right to work. We must realize that AI learns from collective human data, and making human jobs obsolete may also be a moral choice. Unless we are budgeting reskilling and rehiring as a part of corporate strategy, this becomes a challenge.
3. AI systems are environmentally unsustainable. Yes, it is trendy to report that an AI system is net-neutral. However, in reality, it still produces massive amounts of carbon, and off-setting this with existing bio-ecosystems does not neutralize carbon. Furthermore, planting new trees is not the same as an old tree in terms of carbon capture. So we need to ask more questions….and be honest with ourselves.
That is where psychology safety comes from – honesty and transparency and it begins with leadership.
Comments